Biased AI is bad for your health – here’s how to promote algorithmic fairness
AI medical systems promise superhuman capabilities, but they are only as fair as the data they’re trained on.
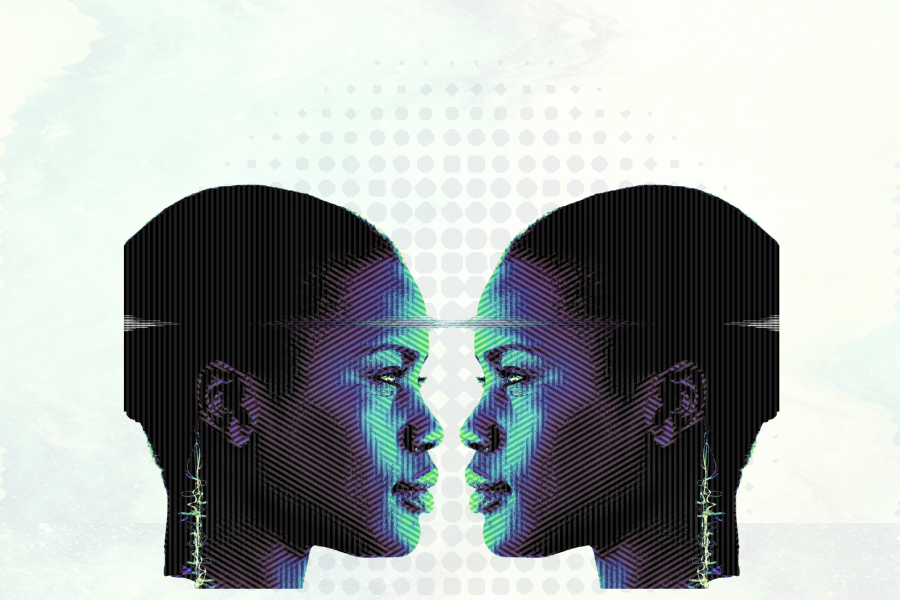
AI medical systems promise superhuman capabilities, but they are only as fair as the data they’re trained on.
This article was written for theconversation.com by Sharona Hoffman, Case Western Reserve University.
Artificial intelligence holds great promise for improving human health by helping doctors make accurate diagnoses and treatment decisions. It can also lead to discrimination that can harm minorities, women and economically disadvantaged people.
The question is, when health care algorithms discriminate, what recourse do people have?
A prominent example of this kind of discrimination is an algorithm used to refer chronically ill patients to programs that care for high-risk patients. A study in 2019 found that the algorithm favored whites over sicker African Americans in selecting patients for these beneficial services. This is because it used past medical expenditures as a proxy for medical needs.
Poverty and difficulty accessing health care often prevent African Americans from spending as much money on health care as others. The algorithm misinterpreted their low spending as indicating they were healthy and deprived them of critically needed support.
As a professor of law and bioethics, I have analyzed this problem and identified ways to address it.
What explains algorithmic bias? Historical discrimination is sometimes embedded in training data, and algorithms learn to perpetuate existing discrimination.
For example, doctors often diagnose angina and heart attacks based on symptoms that men experience more commonly than women. Women are consequently underdiagnosed for heart disease. An algorithm designed to help doctors detect cardiac conditions that is trained on historical diagnostic data could learn to focus on men’s symptoms and not on women’s, which would exacerbate the problem of underdiagnosing women.
Also, AI discrimination can be rooted in erroneous assumptions, as in the case of the high-risk care program algorithm.
In another instance, electronic health records software company Epic built an AI-based tool to help medical offices identify patients who are likely to miss appointments. It enabled clinicians to double-book potential no-show visits to avoid losing income. Because a primary variable for assessing the probability of a no-show was previous missed appointments, the AI disproportionately identified economically disadvantaged people.
These are people who often have problems with transportation, child care and taking time off from work. When they did arrive at appointments, physicians had less time to spend with them because of the double-booking.
Some algorithms explicitly adjust for race. Their developers reviewed clinical data and concluded that generally, African Americans have different health risks and outcomes from others, so they built adjustments into the algorithms with the aim of making the algorithms more accurate.
But the data these adjustments are based on is often outdated, suspect or biased. These algorithms can cause doctors to misdiagnose Black patients and divert resources away from them.
For example, the American Heart Association heart failure risk score, which ranges from 0 to 100, adds 3 points for non-Black patients. It thus identifies non-Black patients as more likely to die of heart disease. Similarly, a kidney stone algorithm adds 3 of 13 points to non-Black patients, thereby assessing them as more likely to have kidney stones. But in both cases the assumptions were wrong. Though these are simple algorithms that are not necessarily incorporated into AI systems, AI developers sometimes make similar assumptions when they develop their algorithms.
Algorithms that adjust for race may be based on inaccurate generalizations and could mislead physicians. Skin color alone does not explain different health risks or outcomes. Instead, differences are often attributable to genetics or socioeconomic factors, which is what algorithms should adjust for.
Furthermore, almost 7% of the population is of mixed ancestry. If algorithms suggest different treatments for Black and non-Black patients, how should doctors treat multiracial patients?
There are several avenues for addressing algorithmic bias: litigation, regulation, legislation and best practices.
AI is becoming more prevalent in health care. AI discrimination is a serious problem that can hurt many patients, and it’s the responsibility of those in the technology and health care fields to recognize and address it.
discrimination are detected and addressed before AI systems receive approval.Algorithmic Accountability Act: In 2019, Senators Cory Booker and Ron Wyden and Rep. Yvette D. Clarke introduced the Algorithmic Accountability Act. In part, it would have required companies to study the algorithms they use, identify bias and correct problems they discover. The bill did not become law, but it paved the path for future legislation that could be more successful.
Make fairer AIs: Medical AI developers and users can prioritize algorithmic fairness. It should be a key element in designing, validating and implementing medical AI systems, and health care providers should keep it in mind when choosing and using these systems.
Sharona Hoffman, Professor of Health Law and Bioethics, Case Western Reserve University
This article is republished from The Conversation under a Creative Commons license. Read the original article.